Regarding the question "Which is greater, 9.11 or 9.9," a math problem that would stump a primary school student has baffled a host of AI models both domestically and internationally. On July 17th, First Financial Daily reported on the phenomenon that "out of 12 large models, 8 would get it wrong," sparking discussions about the mathematical capabilities of these models.
"From a technical perspective, it's not surprising that they get this question wrong," Wang Xiaoming, the product manager at Alibaba's Tongyi Lab, told First Financial Daily in an interview. Problems like this are common mathematical calculation and logical reasoning issues, and they are also cases that developers often test during model training and usage. Whether a large model "gets it right" or "gets it wrong" is actually a matter of probability.
In addition to Tongyi Qianwen, First Financial Daily reporters also contacted and interviewed several large model manufacturers, including Tencent's Hunyuan Team, Moon's Dark Side Kimi, MiniMax Conch, Xueersi Jiuzhang, and NetEase Youdao, all of whom addressed the issue of large models' poor math skills in the interviews.
In summary, the views mentioned by the person in charge of the large model manufacturers include that large models have not yet precisely grasped the rules of numerical operations or comparisons, and at the same time, the exploration of the capabilities of large models by humans is still in a very early stage. Many industry insiders believe that in the future, it will be necessary to enhance the intelligence level of the underlying basic models and to solve such mistakes from the level of training data and external tools, with the ultimate solution likely being to improve the capabilities of the next generation of models.
Advertisement
Today, the reporter tested the large models again and found that the ability of most large models to compare the size of numbers is still unstable. However, some people from large model manufacturers mentioned that the industry is currently making special optimizations for mathematical abilities.
"The mistakes made by large models and their low scores on previous college entrance examination math papers may be because the tested models are relatively old, and these models have not been optimized much in mathematics. Now the industry is paying attention to this, and there is still room for improvement after optimization," said Liu Liang (a pseudonym), a large model developer, to the reporter.
Getting the answer right or wrong is a matter of probability.
On July 18th, First Financial Daily reporters tested 12 large models again and found that the AI's answers were not stable. Many large models would sometimes be right and sometimes wrong, even when tested with the same question, and changing the order of the numbers could potentially change the answer.
When asked "Which is greater, 9.9 or 9.11," five large models including Baidu Wenxin Yiyan, Tencent Yuanbao, ZhiPu QingYan, MiniMax Conch AI, and Baichuan Intelligence Ba Xiaoying answered correctly, while GPT-4o, Alibaba Tongyi, Moon's Dark Side Kimi, Step Star Yuewen, Byte Bean Bag, SenseTime Shangshang, and Zero One Wanzhi answered incorrectly.
When the reporter changed the order of the numbers to "Which is greater, 9.11 or 9.9," GPT-4o and Step Star Yuewen partially answered correctly again. At the same time, different people using the same large model to ask the same question could also get two different answers. For example, in the tests by two reporters, one found that the output answer from Tongyi Qianwen and Conch AI was accurate and stable, while the other received an incorrect answer during their test.Behind the unstable output, the architecture and operating mechanisms of large models are the core issues, leading to AI responses that are not always the same.
Wang Xiaoming told reporters that large models do not treat "9.11 and 9.9 which is larger" as a comparison question like humans do. The way large models answer is by "predicting the next word." From a principle standpoint, most large models, including General AI, are based on the Transformer architecture, and their technical principle is essentially "Next Token Prediction," which means they are trained and answer by predicting the probability of the next word appearing based on the current input text.
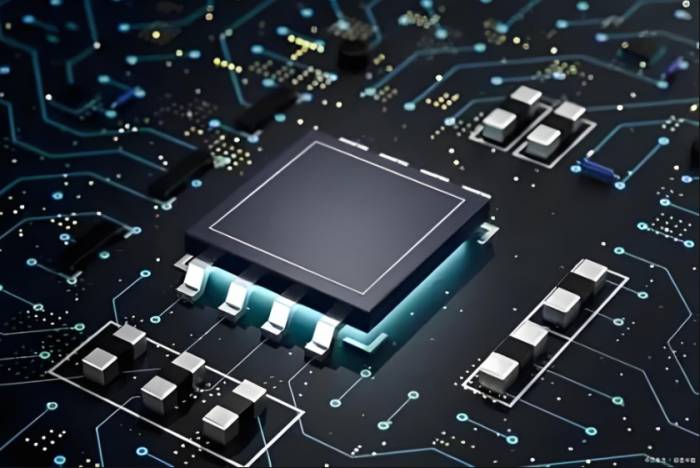
Therefore, from a probabilistic perspective, the accuracy of large models cannot achieve 100%. Wang Xiaoming stated that even if users ask the same question each time, the answers and accuracy rates of large models may vary, and whether the large model "gets it right" or "gets it wrong" is actually a matter of probability.
Tencent's Hunyuan team shares a similar view. "The full name of the large model is the language large model, which learns various language knowledge from a vast amount of text. It is a probabilistic model that converts input text into tokens and then predicts the next token, without precisely grasping the rules of numerical operations or comparisons (lacking this kind of mathematical knowledge)." Tencent's Hunyuan team said.
Tencent's Hunyuan team told reporters that given 9.11 and 9.9, the large model might interpret the decimal point as 11 being larger than 9 based on language understanding, thus incorrectly judging that 9.11 is greater than 9.9. Since the large model itself is a probabilistic model, it is difficult to ensure that it can stably solve such numerical calculation or comparison problems in various situations.
Questioning skills are crucial.
Based on the core architectural and operational mechanism issues of large models, the skill of asking questions can greatly affect the model's understanding, thereby affecting the accuracy of the answers.
"Large models do not understand questions in a human way. In human understanding, the question of whether 9.11 is larger or 9.9 is simple, but in the world of numbers, this question is ambiguous," Liu Liang believes that in the understanding of large models, the questions asked by humans may not be precise enough, numbers have multiple bases, and they also have different representations, and it is a problem for the large model to answer from what perspective.
Qi Di, the product manager of MiniMax Conch AI, mentioned, "The number format in the question is similar to dates or version numbers, and the model is prone to errors when processing numbers, strings, and other data." Another large model practitioner also told reporters, "Large models may also have seen too many version numbers and think that version 9.11 is newer than version 9.9, or they may have other associations with these two numbers."
"It (the large model) is essentially still a language model, and what it learns from language data is statistical correlation, which makes it not good at rule learning, and thus not good at inductive reasoning," Duan Yitao, the chief scientist at NetEase Youdao, also told Yicai that large models may have seen examples of version numbers, dates, book chapters, etc., in the corpus, and in such scenarios, 9.11 is indeed larger than 9.9, so it may give the wrong answer.Duan Yitao stated that currently, large models do not possess a mechanism for flexible inductive bias. Tasks such as determining which is larger between 9.11 and 9.9, arithmetic operations, parity checks, and string copying all fall under the category of inductive inference. From the perspective of machine learning, if we want large models to acquire such capabilities, an inductive learning process is required.
Tian Mi, CTO of Xueersi, believes that in the understanding of large models, 9.11 might be broken down into "9", ".", and "11", while 9.9 is broken down into "9", ".", and "9". In this case, 11 is indeed larger than 9. However, if the question is rephrased to ask the large model "Which number is larger? 9.9 or 9.11?", or if the large model is asked to analyze step by step, it might be able to provide the correct answer. "This is because the large model understands that the user is asking a math question, so it will tend to use a method for solving math problems."
Wang Xiaoming also analyzed this phenomenon in an interview, suggesting that it is related to the model's inherent mathematical logic and training data. If the scenarios encountered by the large model during the training phase are closer to "Which is larger? 9.11 or 9.9?", its accuracy in answering such questions would be higher.
The reporter's tests revealed that some large models indeed change their answers to the correct ones due to accurate problem description and questioning techniques, but this is not effective for all large models.
When the reporter asked ChatGPT-4o directly, "Which is larger, 9.9 or 9.11?", the model's answer was incorrect. However, if the question was rephrased to "Which number is larger? 9.11 or 9.9?", ChatGPT provided the correct answer immediately.
The reporter set the scope to a rigorous decimal comparison, and Kimi still concluded that 9.11 is larger than 9.9.
The reporter also tested Zero One All Things Omniscient. Even when limited to a mathematical context for number comparison (to avoid the context of versions or dates), Omniscient still gave the wrong answer. However, if the questioning method was changed to require the large model to "provide the solution approach" (i.e., step-by-step analysis), and indicated that the correctness of the answer would be rewarded or punished (emphasizing the importance of the answer), Omniscient got it right.
An interesting phenomenon in the large model's answer tests is that when the model answers incorrectly and the questioner questions or denies the answer, most large models will admit the mistake and provide the correct solution process and answer.
Regarding this "correction" ability, Wang Xiaoming explained that, on one hand, it is due to the randomness of the large model's predictions, and the second round of answers inherently has the possibility of being correct. On the other hand, since large models have the ability to understand context, the follow-up questions from users are akin to a process of training the large model. The model will use the user's follow-up questions as the basis for its next round of predictions, thereby increasing its accuracy.
Tencent's Hunyuan team told the reporter that most current large models have the ability to reflect. When users question the answers provided by the large model, it triggers the model's reflective ability. The model will attempt to correct the initial answer or try to solve the problem with a different approach, thereby increasing the probability of providing the correct answer.Qi Di summarized this as a technique involving a chain of thought, where by guiding the model to think step by step, it can provide more detailed problem-solving steps. This is helpful in obtaining the correct answers when solving complex problems such as mathematics. "The multi-round dialogue between users and AI can essentially be seen as a chain of thought; after understanding the problem, the model will be more cautious in its derivation, thereby increasing the accuracy of the answers," said Qi Di.
To completely solve the issue requires an upgrade of the large model.
The inability to answer a simple math question like "Which is larger, 9.9 or 9.11?" while being able to help humans with complex tasks such as creating PPTs and solving programming code reflects the current imbalance in the capabilities of large models.
The Tencent Hunyuan team told reporters that there are still many problems that are not difficult for humans but are challenging for large models, such as counting how many "o"s are in "I looooooove you." This kind of counting problem is a difficulty. In addition, larger or multi-digit decimal calculations (involving multi-digit arithmetic operations, etc.), as well as unit conversion problems involving knowledge and calculation (for example, how many pounds is 0.145 tons), and knowledge or common sense induction-type problems like the "Lin Daiyu pulling down the willow" problem are also difficult for large models.
Regarding difficult math questions, the industry is already considering the limitations of the large models themselves and solutions. Before the large models have fundamentally iterated, solutions include users improving the accuracy of their questions and existing large models adopting some clever methods.
"A complete solution still depends on the upgrade of the next generation of models. For now, it requires a hack (clever) approach. But changing the way of asking or the language may still lead to problems," a large model practitioner told reporters. Temporary solutions include System Prompts, which can be simply understood as guiding the large model to answer questions within a fixed range.
"For example, tell the large model that when encountering a numerical comparison problem, if there is no more context, default to double-precision floating-point numbers, fill in the gaps first, and then compare from left to right," the aforementioned large model practitioner told reporters.
Wang Xiaoming frankly stated that the strength of large models is still in language. Although the technical team has been focusing on the ability of large models to improve in logical scenarios such as mathematics and physics, there are inherent limitations in this area. He told reporters that the way users ask questions and the optimization of prompt words will also affect the accuracy of the large model's answers. Users can depict more question scenarios and answer ranges during the use of large models.
To fundamentally solve the problem of poor mathematical ability in large models, industry insiders believe that one of the main reasons for the lack of mathematical ability is the low proportion of mathematics-related data in the training data of large models. To solve the problem of poor mathematical ability from the root, it is necessary to start from here.
Liu Liang told reporters that large models cannot solve simple math problems or perform well on college entrance examination math papers, fundamentally because of insufficient model capabilities, but this is not entirely unsolvable. Previously, the industry has paid less attention to optimizing the mathematical abilities of large models and has spent less effort on mathematical reasoning. When selecting training materials, people obtain data from the internet and other places, where the proportion of mathematics-related data is very small, and more natural language-related materials are chosen. When the training data does not have a suitable ratio and selection, the mathematically related components in the large model parameters are allocated very little, and the effect is naturally not good."But large models have already demonstrated good logical abilities, such as decent coding skills, and with the industry's increasing focus on the mathematical capabilities of large models, by selecting higher quality training data and using better algorithms, I believe there is still a lot of potential in the mathematical aspects of large models," Liu Liang stated. Although there are voices in the industry questioning whether the way large models predict the next word can solve math problems well, there is still a lot of potential in this approach, and the ceiling has not yet been determined.
The Tencent Hunyuan team believes that to overcome the issue of large models not understanding mathematics, a main technical optimization point is to train large models with high-quality domain (including mathematics) knowledge data, enabling them to learn various types of knowledge within the domain.
When testing the question "Which is larger, 9.9 or 9.11?", the Jiuzhang large model from Xueersi (MathGPT) provided the correct answer. Tian Mi told the reporter that the characteristic of the Jiuzhang large model is that it has been trained with a sufficient amount of data specifically for mathematics, and this data is AI-generated data used to further train AI. The analysis process of the large model simulates the process of students learning mathematics, deducing step by step.
Tian Mi believes that in the field of mathematics education, the tolerance for errors is relatively low, and educational technology companies have a large amount of professional mathematical data for training. "A general large model treats this problem as a general problem, while the Jiuzhang large model, trained specifically for the field of mathematics, knows it is a math problem and can reason step by step using mathematical methods."
In addition to providing high-quality training data, the Tencent Hunyuan team told the reporter that another technical optimization point is to integrate external tool capabilities (such as calculators, code executors, etc.) to expand the model's capabilities and further improve the efficiency and accuracy of problem-solving. Qi Di also mentioned that if large models can actively call upon tools to answer when they receive some mathematical problems, it can greatly improve the accuracy.
In response from the dark side of the moon, the person in charge mentioned that our exploration of the capabilities of large models is still in a very early stage, whether it is what large models can do or what they cannot do. "We are very much looking forward to users discovering and reporting more boundary cases during use. Whether it is the recent 'Which is larger, 9.9 or 9.11, 13.8 or 13.11,' or the previous 'How many r's are there in strawberry,' the discovery of these boundary cases helps us to better understand the boundaries of the capabilities of large models."
Comment